DATA MANAGEMENT: TRANSFORMING DATA INTO USEFUL INFORMATION
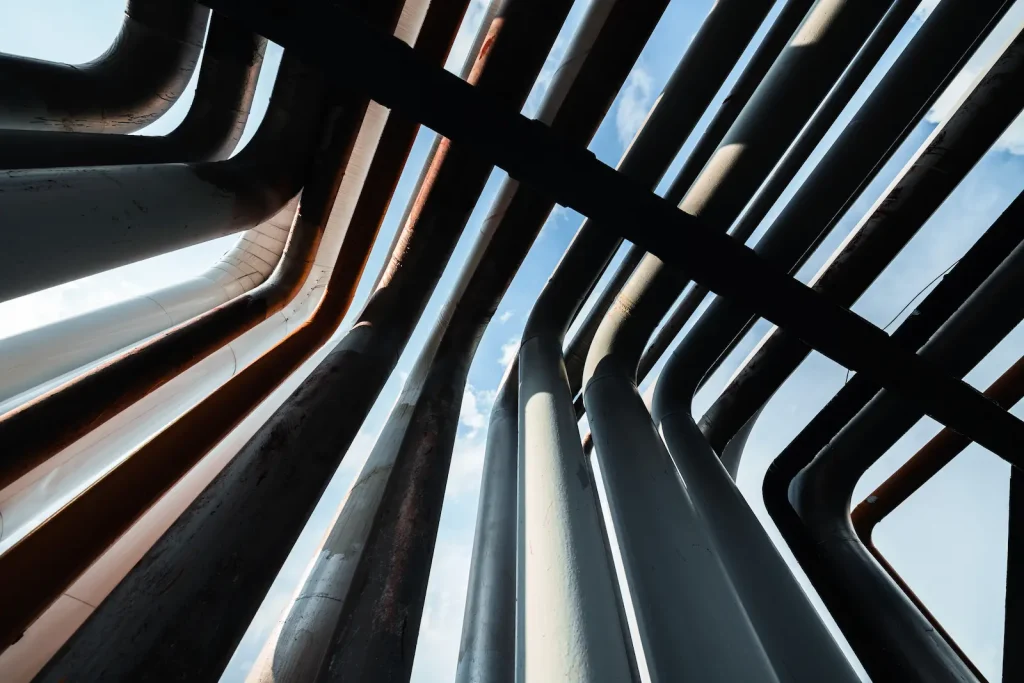
COLLECT, PREPARE, ANALYZE, AND DELIVER
Data is a key component for an increasing number of use cases, to the point where a use case-centric approach no longer meets the needs for flexibility and time-to–market.
Transforming raw data into gold data tailored to your business
Aggregate then cluster according to each objective: analytics, operations, ML, and data science…
Consume customized, reliable, and traceable data in dedicated spaces.
Data is a key component for an increasing number of use cases, to the point where a use case-centric approach no longer meets the needs for flexibility and time-to-market. A shift towards a more open approach is necessary: providing consumable data to different stakeholders without presupposing use cases, which evolve over time. Data becomes a product whose consumption is the responsibility of the services that produce it.
In this context, it is no longer sufficient to simply circulate data, but to give it meaning that adds value. Hence the need for a dedicated ecosystem, combining integration and platform tools with functions for formatting and quality control.
This ecosystem has introduced the notion of a data pipeline to encompass all the steps from collection to sharing of data, directly consumed by operational teams.
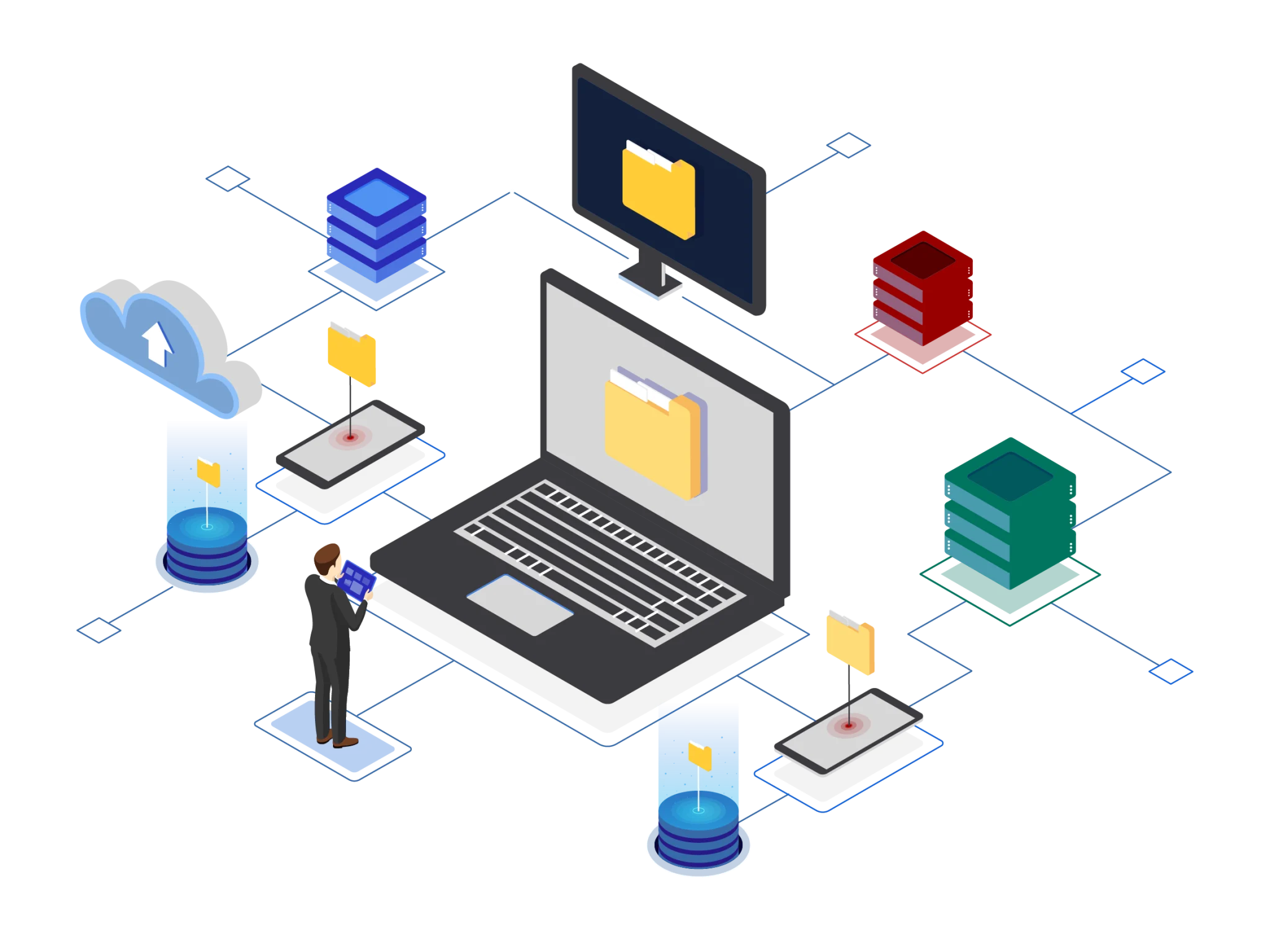
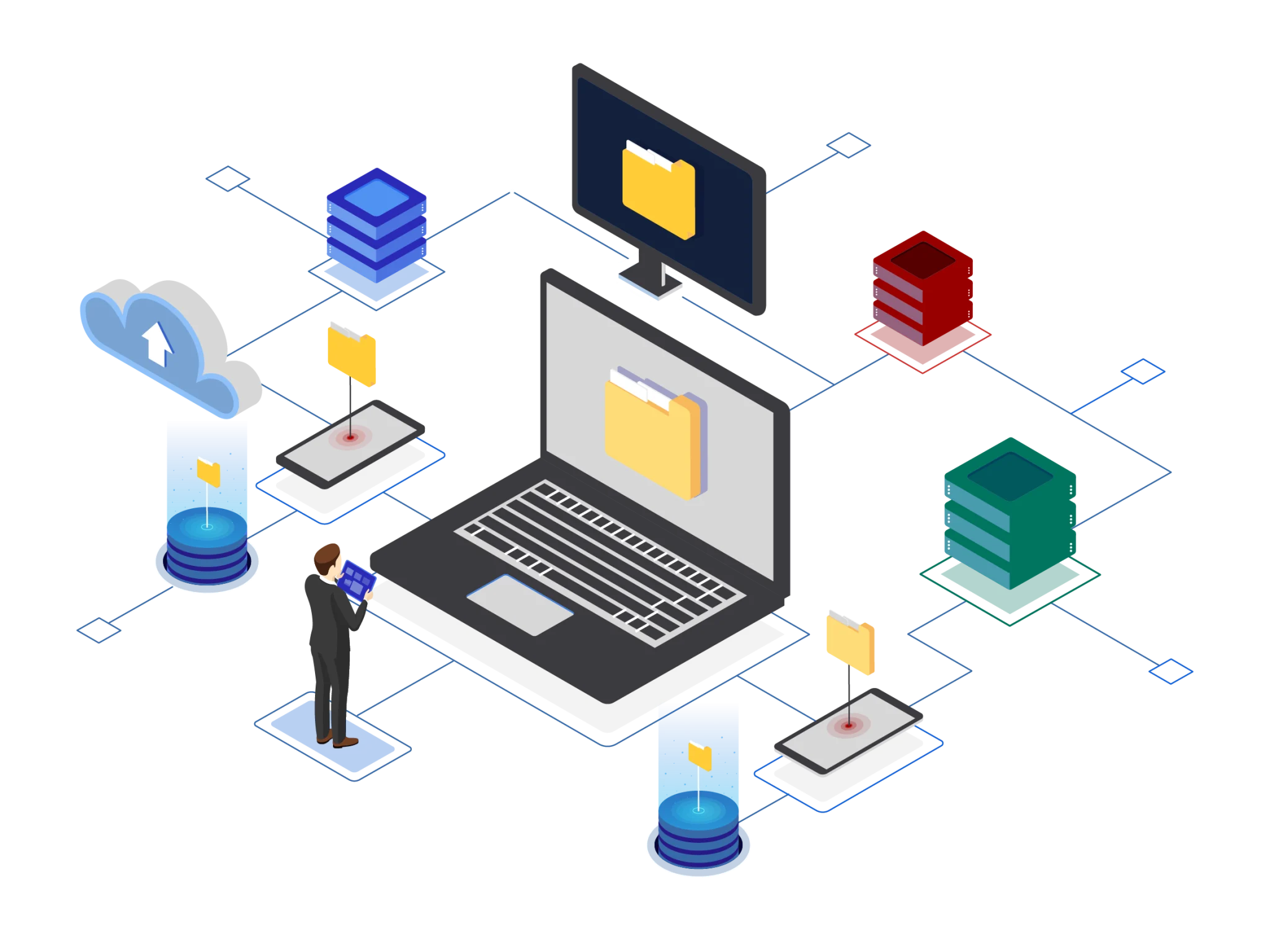
Data is a key component for an increasing number of use cases, to the point where a use case-centric approach no longer meets the needs for flexibility and time-to-market. A shift towards a more open approach is necessary: providing consumable data to different stakeholders without presupposing use cases, which evolve over time. Data becomes a product whose consumption is the responsibility of the services that produce it.
In this context, it is no longer sufficient to simply circulate data, but to give it meaning that adds value. Hence the need for a dedicated ecosystem, combining integration and platform tools with functions for formatting and quality control.
This ecosystem has introduced the notion of a data pipeline to encompass all the steps from collection to sharing of data, directly consumed by operational teams.
However, there is no single architecture that meets all requirements.
At least three elements are key:
- Type of data: For example, reference data can be managed specifically in Master Data Management (MDM) systems. Similarly, real-time individual data is handled differently than population data, which requires sequential processing over time.
- Data volumes: For example, the number of asset records is vastly different from the volume of transactions. Moreover, B2B services are not comparable to in-store sales of thousands of different items.
- Refresh requirements: Analytical needs often rely on cold data, while customer journey orchestration depends on event-driven logic using hot data.
For businesses, data management has become a crucial challenge, combining business expectations, scalability, security, and cost control. The pooling of data management tools based on their roles, within a data platformization, allows mastering the technology, building a 360° view, and enhancing operational efficiency to provide successful user experiences, both internally and externally. This contributes to performance while offering the necessary flexibility in the short and medium term.
DATA PROCESSING
Data management sits between data governance, which defines the principles (roles, rules, etc.), and the architecture that supports its implementation. This can be grouped under the term data processing, with the following principles:
This can be grouped under the term data processing, with the following principles:
- Include transformation functions:
- Make raw data queryable (via SQL, Python, etc.), regardless of its initial format: structured, semi-structured, or unstructured.
- Align metadata with the company's glossary, as it appears in the Data Catalog.
- Ensure data quality: remove noise, standardize, apply business rules, deduplicate, enrich with external providers, etc.
- Store data to facilitate access and processing:
- Use storage media and formats appropriate to the expected volumes and availability.
- Adopt a model that facilitates access and query performance.
- Partition by domain or workspace to optimize compute performance.
- Make data available for different objectives:
- Analytics: Prepare data and create metrics used for reporting, dashboards, and any form of visualization.
- Operational: Create specific data for each type of actor and make it accessible, including allowing them to build their own metadata, such as complementary indicators.
- ML and Data Science: Provide as broad a data set as possible to enable experts to achieve the best certainty rate.
- Data sharing: Offer data in a queryable format, with a marketplace logic defining who has access to what.
DATA CONTROL AND SECURITY
Once data pipelines are in place, additional needs arise to control data transformation and ensure secure sharing.
Among these needs, at least three are worth mentioning:
- Observability: As systems become more complex, maintaining their performance and reliability becomes a challenge. This is where monitoring and observability capabilities play a crucial role. Neglecting this aspect exposes the company to multiple failures and their potential impacts.
- Lineage: This involves improving data knowledge to better analyze and control the impacts, whether intended or not, while meeting a regulatory objective: being able to justify the origin of every piece of data.
- Anonymization, pseudonymization, data masking: Legal constraints (particularly on sensitive data) reinforce the need to control who has the right to see what, and at what level of granularity.